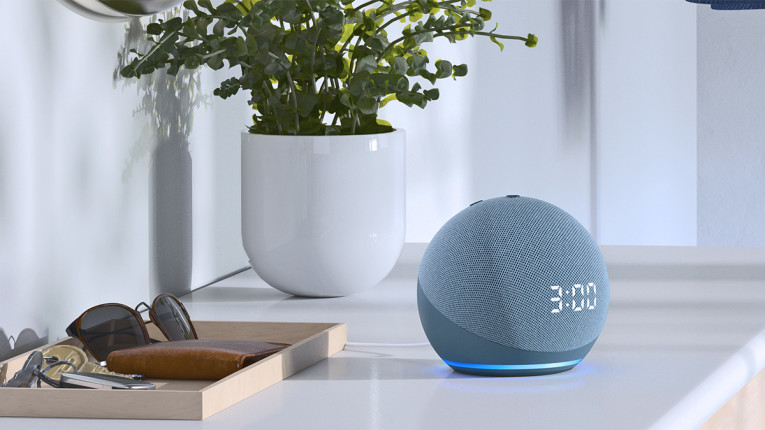
In recent years, voice technology has proliferated the market, with more devices becoming enabled with speech recognition for added convenience to the end user. Most popularly, smart speakers have dominated the voice recognition market, with more than 157 million smart speakers in homes across the US. [1]
Since this success, speech technology is beginning to pop up in other areas, including home appliances, vehicles and more. However, key areas of the market remain untapped, as applications of speech technology are far wider than just smart home devices.
With this in mind, Fluent.ai, an embedded voice recognition solutions company, has developed a technology that operates fully offline. The company was founded more than 5 years ago based on the research done for my PhD thesis on deep learning based speech recognition.
Today, Fluent.ai’s solution offers a great deal of features that address common problem areas with speech technology, including being multilingual, able to understand any language and accent, operating fully offline, offering another layer of privacy, and more. The technology utilizes a unique speech-to-intent model, bypassing the need for the traditional speech-to-text approach. This allows the solution to operate fully offline, never needing to transmit information to the cloud, and therefore offering a more private solution. Figure 1 compares conventional speech models to that of Fluent.ai.
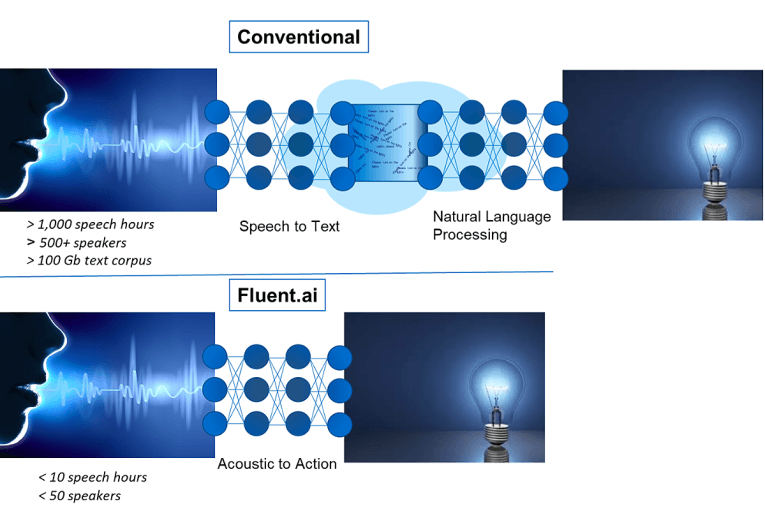
Possible Applications
Fluent.ai primarily works with original equipment manufacturers (OEMs) and original device manufacturers (ODMs) to embed its technology in a variety of products, working within industries such as manufacturing, retail, smart home, and more. Applications of the technology run the gamut, as Fluent.ai’s technology is made to be nimble and applicable for everything from wearables to robotics (Figure 2).
This year, Fluent.ai attended the all-digital CES, and introduced a Speaker ID function with its technology. This enables the tech to provide personalized responses to a user depending on who speaks the command. For example, if two different users ask a device to play their favorite music, two different kinds of music will play based on what it knows about each individual’s preferences.
Today’s consumers are looking for increasingly personalized experiences, and interacting with their technology is no exception. This has led to an increasing demand for speaker ID tech to be integrated into more household items. Fluent.ai’s offerings are making strides over the hurdles of traditional speech technology, creating a robust solution in today’s market that overcomes many challenges.
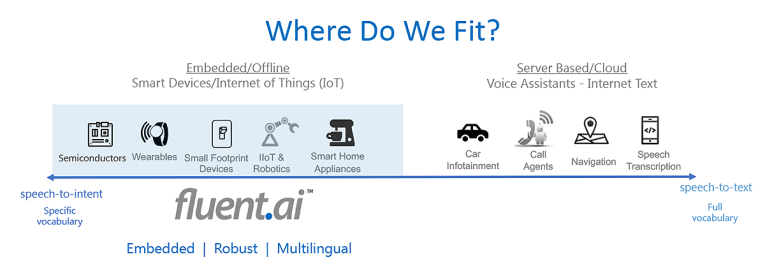
Profiling Voices
In today’s economy, consumers are continuing to look for more personalized experiences — with more than 80% of people indicating they’re more likely to make a purchase when offered a personalized experience. [2] This same desire and mentality can be applied across industries. With this in mind, personalized technology is also on the rise. Consumers are continuing to hunt for technology that understands their personal preferences and reacts accordingly.
Speech technology is no exception. Speaker ID has recently risen in popularity, with major players such as Apple and Samsung beginning to scratch the surface of this. However, developing an offering such as speaker ID doesn’t come without its hurdles.
One of the greatest challenges for speaker ID technology is ensuring that the device responds accordingly to each unique user’s requests. This requires detailed efforts to ensure that each voice is correctly profiled to respond appropriately. All of Fluent.ai’s products are based on cutting-edge neural network technologies. For training the models, a large number of audio files are used from different speakers — spanning across languages, dialects, accents, and more. From this, the model learns the voice profile of a user from just a few utterances.
Once the profile is established, the neural network is able to leverage this model in order to provide personalized responses. As an added benefit, because the models are initially trained on data recorded through a variety of different front ends, these models do not have a dependency on any particular signal processing front end. Furthermore, the registration of a new user would happen on the final device that the technology is embedded on, therefore the registration would be done on the front end of this device, as opposed to the software.
Low Power
While it may not be immediately top of mind for a consumer, low-power solutions are extremely beneficial. This isn’t something an end user often thinks about, until they find the battery of their device quickly dies, ultimately leading to a great deal of frustration. This makes it imperative for OEMs to seek out low-power solutions to help ensure satisfaction from their end users, no matter the industry.
For artificial intelligence solutions, the latest edge technology is all about consuming the least amount of power while creating a solution that is optimized for peak performance. Furthermore, a low-power solution is more cost-effective, making it more attractive to utilize. Fluent.ai’s products are always developed to run on low power, and its speaker ID is no exception. This enables the technology to be nimbler and more applicable across industries — previously a pain point of adoption. [3]
The speaker ID model works in conjunction with Fluent.ai’s wakeword model. For example, when a user speaks the programmed wakeword, such as “computer,” the system recognizes if the correct wakeword is being spoken by a known or registered user. To maintain a low-power solution, the team designed a neural network architecture in which in order to achieve parallel recognition of both the wakeword and speaker ID simultaneously. By layering these models together, the software is able to operate with less computing power because they can draw upon similar models to achieve the goal of responding to a command.
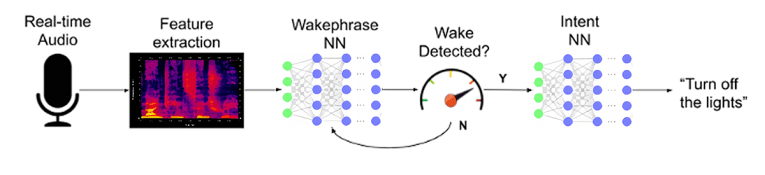
AI on the edge has been a hot button topic lately, with adoption accelerating over the last few years. As artificial intelligence continues to touch nearly every industry in some capacity today, edge processing is beginning to proliferate too. Fluent.ai uses a series of steps to optimize the neural networks for edge processing.
Its proprietary neural network architectures use less computing power as compared to the industry. Furthermore, the team utilizes several neural compression and mathematical optimization techniques, which reduce the size and computing requirements of Fluent.ai’s networks. And, Fluent.ai has its own runtime library — Fluent μCore — that is optimized for an increasing number of hardware platforms.
This enables the company’s technology to have even greater applications. The Fluent μCore is able to perform streaming neural processing, which results in smaller memory requirements, and therefore it can run more capable networks on memory and CPU-constrained edge devices.
With all of this in mind, Fluent.ai leveraged edge processing to help develop its speaker ID technology. This lent itself to creating the neural network architecture that enabled the company to layer the wakeword and speaker ID models, and ultimately create an efficient and low power solution (Figure 3).
Multilingual
Currently, there are more than 6,500 languages spoken worldwide. However, there are still many hurdles that mainstream technology needs to overcome to be more accessible to greater populations.
Within speech technology, being understood is one of the greatest pain points for an end user. Mainstream options still have trouble understanding stutters and accents, as well as bilingual users and voices of people of color. While the industry is working to address these challenges, they are typically retroactive solutions, instead of solutions that are built into the technology at the start.
Similar challenges can be found in speaker ID technology, but Fluent.ai’s approach ensures that this tech is more widely accessible. All of the company’s offerings are trained to understand hundreds of languages, accents, speech impediments, and more, ensuring that the solution will respond accurately no matter the speaker’s background. Fluent.ai’s speaker ID solution is no exception, specifically programmed with its technology that can recognize any language, accent, impediment, and more. As the demand for more personalized user experiences rises, the call for technology to be more inclusive will also rise.
While the company only recently introduced the tech at CES 2020, Fluent.ai’s speaker ID technology has a variety of potential consumer applications, including everything from system preferences in business meeting rooms, to playing a user’s favorite music on their smart home device. As end users continue to look for personalized solutions, speaker ID technology will continue to rise in popularity.
Through Fluent.ai’s technology, OEMs can help deliver on this demand by integrating the embedded solution into their offerings. aX
This article was originally published in audioXpress, April 2021.
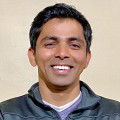
Vikrant Singh Tomar is the founder and CTO of Fluent.ai, a speech recognition company based in Montreal, Canada. Vikrant has more than a decade of experience in speech recognition and machine learning, and holds a PhD in automatic speech recognition and machine learning from McGill University. He has worked at Nuance Communications and Vestec Inc. in the past.
References
[1] “The Smart Audio Report,” NPR and Edison Research, February 2020,
www.nationalpublicmedia.com/uploads/2020/04/The-Smart-Audio-Report_Spring-2020.pdf
[2] “The Power of Me: The Impact of Personalization on Marketing Performance,” Epsilon, January 2018,
[3] B. Bailey, “Power Is limiting Machine Learning Deployments,” Semiconductor Engineering, July 2019,