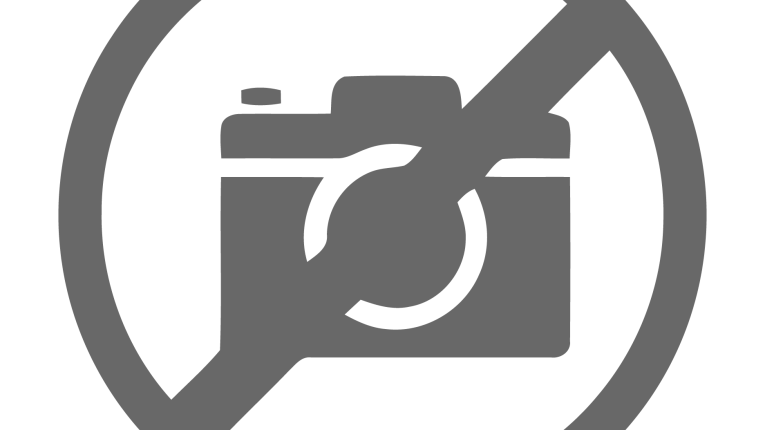
Earphones with an Open-ear True Wireless Stereo (OTWS) form factor have rapidly gained popularity, with all major audio brands now including such products in their portfolios. Open-ear designs offer unprecedented comfort and cater to evolving user behaviors — particularly among younger consumers who wear earphones all day while staying connected to their surroundings.
This rise is particularly noteworthy given the latest focus in the hearable industry on delivering high audio quality in any environment and improving ANC performance. Both are more challenging to achieve in open-ear designs, as freeing the ear canal from the silicon tip creates significant obstacles in balancing sound performance and insulation.
Consequently, the challenge of balancing comfort and noise attenuation has long been viewed as insurmountable, as open designs allow sound to leak in, and traditional ANC technology is less effective in non-occluded ears. However, in recent months, two brands have proven that it is possible to meet this challenge using distinct approaches: first Apple with its AirPods 4 ANC loose-fit design and more recently Cleer with its ARC III Pro open-ear earphones. Two different implementations in different form factors, showing that ANC is also possible in non-occluded designs.
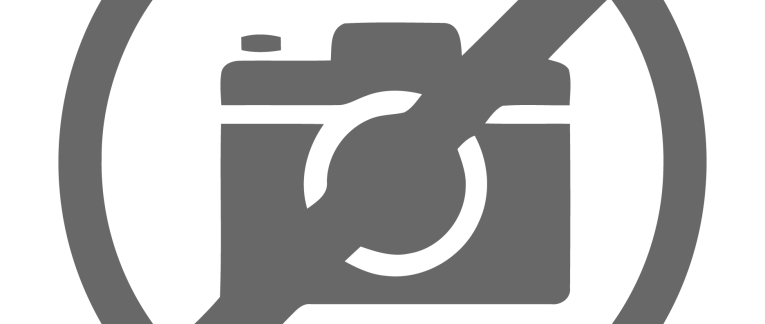
Historically, ANC was developed with a singular goal: to block out external noise as effectively as possible, providing users with a serene, undisturbed listening experience. This was achieved through occluded designs, which offered the best possible passive insulation and constant pressure, creating a predictable environment for ANC. Today, many audio brands have mastered ANC in these occluded designs, delivering up to 35dB of attenuation across the frequency spectrum.
However, audio leaders such as Apple and Cleer have introduced a new paradigm by incorporating ANC technology into, respectively, loose-fit and open-ear hearables — devices intentionally designed to allow some external sounds through.
This shift raises an important question: Why are companies developing ANC for designs that don’t prioritize total isolation? The answer may lie in the quest for a new balance between physical comfort (avoiding the intrusive feeling associated with in-ear devices) and sound comfort (reducing exposure to tiring or annoying background noise from their surroundings). Figure 1 illustrates the differences among in-ear, loose-ear, and open-ear designs.
Currently, the challenges related to ANC revolve around achieving in-ear ANC performance in loose-fit designs while inventing a new approach for open-ear designs, which are inherently meant to be open to the world.
Overcoming Design-Specific ANC Challenges
Acoustic consistency in loose-fit and open-ear designs.
In traditional occluded designs, passive attenuation typically provides around 20dB per decade starting between 100Hz and 500Hz. In contrast, a loose-fit design offers attenuation only above 5kHz, while open-ear designs provide almost none. In occluded designs, passive attenuation plays a central role in canceling frequencies above 1kHz, where active systems are less effective. The passive attenuation thus serves as a “low-pass filter” for external noise, introducing additional group delay between reference and control microphones, which, in turn, enables the ANC system to maintain a certain causality between the primary noise and its anti-noise response.
To maintain consistency in acoustic performance, manufacturers often introduce controlled acoustic leakages between the speaker’s front cavity and the external environment. This approach mitigates the impact of unpredictable, minor leaks that can occur at the interface between the ear tip and the ear canal. It also helps to stabilize both the passive attenuation and speaker response across users.
In loose-fit and open-ear designs, various factors can significantly influence the acoustic of the device. With minimal passive insulation, variations in the device placement inside the pinnae, due to user-specific factors such as morphology or usage habit, can alter the speaker frequency response (secondary path) and the transfer function between reference to control mic (primary path), necessitating real-time filter adjustments.
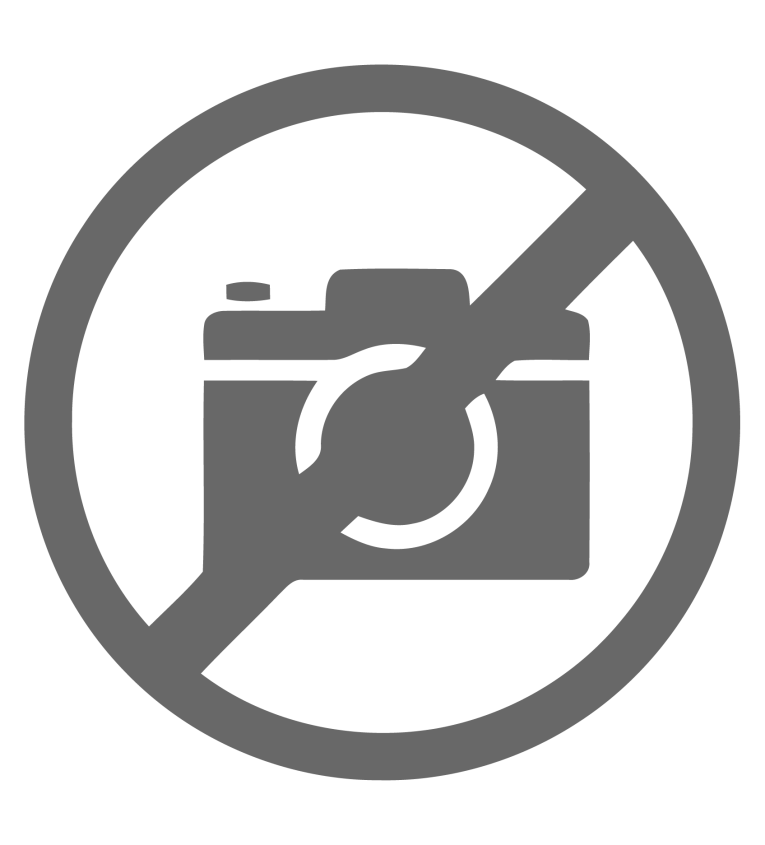
ANC systems are often likened to a jet powered by a reaction engine, where the propulsion is located behind the center of mass; any misalignment in the propulsion direction causes the jet to roll over. In earphones, instability results in howling, characterized as a high-pitched sound from a feedback loop when microphones pick up sounds emitted by the speakers.
For this reason, traditional ANC design focuses on optimizing attenuation performance while maintaining stability margins in gain and phase. These margins account for variability between products and use-cases. To mitigate the impact of these variations, adaptive techniques have been added to the traditionally used second-order recursive linear filters.
In open-ear and loose-fit designs, howling often occurs when the system experiences sudden shifts in acoustic conditions—such as when users bring their hands near the device to press a button. This proximity can unintentionally intensify feedback loops between the speaker and microphones. Traditional adaptive ANC approaches are insufficient to ensure system stability in these scenarios, so howling detection techniques are necessary to continuously monitor stability and prevent unwanted Larsen effects.
Using AI to detect and monitor howling.
As a high-gain closed-loop system with highly variable externalities, the system’s stability margin must be closely monitored and adjusted quickly to prevent howling from becoming noticeable to the user (Figure 2). Achieving this goal of sub-millisecond latency for adaptation poses a challenge that statistical algorithms and classic DSPs struggle to meet. That scenario is a prime example of how neural networks, powered by new generations of ultra-low latency processors, are capable of changing the game.
AI-ANC technologies can monitor the howling margin every millisecond and dynamically trigger the re-parameterization of the ANC depending on the context. This adaptability allows the system to optimize performance through real-time responses to changes in the acoustic environment and enhances predictive capabilities by anticipating potential instability conditions based on learned data.

Open-ear designs amplify the challenges already present in loose-fit ANC models, requiring even more sophisticated solutions. While loose-fit models allow some external sound to enter, open-ear designs are intentionally constructed to leave the ear canal fully open, which introduces a unique set of difficulties for noise cancellation technology.
In open-ear configurations, the speaker is positioned much farther from the ear canal than in-ear or loose-fit designs, introducing a substantial group delay between the speaker (control source) and the ear canal (control zone). This added delay challenges the system’s ability to maintain causality, thereby reducing noise cancellation effectiveness, especially at higher frequencies.
Virtual sensing algorithms for active noise control.
Unlike in-ear and loose-fit designs, where a microphone near the ear canal (feed-back microphone) can approximate the ANC effect at the eardrum, open-ear designs prioritize unobstructed ear access, making it impractical to place control sensors near the ear canal. Consequently, control signals cannot be fed back into the system directly, creating limitations in achieving high-performance noise cancellation.
To address this last challenge, virtual sensing algorithms have been developed for ANC (Figure 3). These algorithms utilize the physical error signal, the control signal, and knowledge of the system to estimate the error signal at a remote location, referred to as the virtual location [1].
The block diagram shown in Figure 4 illustrates an Internal Model Control (IMC) adaptive virtual microphone control system (VMC) designed for an active headrest.
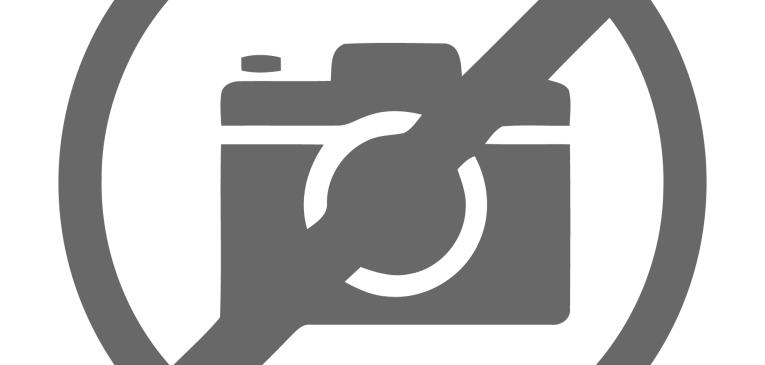

In this algorithm, the signal measured at the control microphone position (y(i)) is not used at the error of the Least Mean Squares (LMS) method, as is typical in classic noise-canceling algorithms. Instead, the error is a combination of the physical error (y(i)) and the control algorithm input (x(i)), convoluted by an appropriate auxiliary filter H (AF) [2].
This filter H is specifically designed to estimate the error signal at the eardrum position and is trained during a tuning stage with a physical microphone placed in the intended control zone (Figure 5), using an artificial ear or Head-and-Torso Simulator (HATS), to optimize noise-canceling performance.
Such approaches enable remote optimization of noise cancellation from the control microphone’s location. However, the effectiveness of these techniques is highly dependent on the accuracy of the aforementioned estimators. These estimators are developed and optimized in controlled tuning environments, which may not accurately reflect real-world usage conditions. As a result, the auxiliary filter and secondary path estimators may be suboptimal, potentially leading to poor ANC performance or even system instability (e.g., Larsen effects). Consequently, these metrics require dynamic optimization.
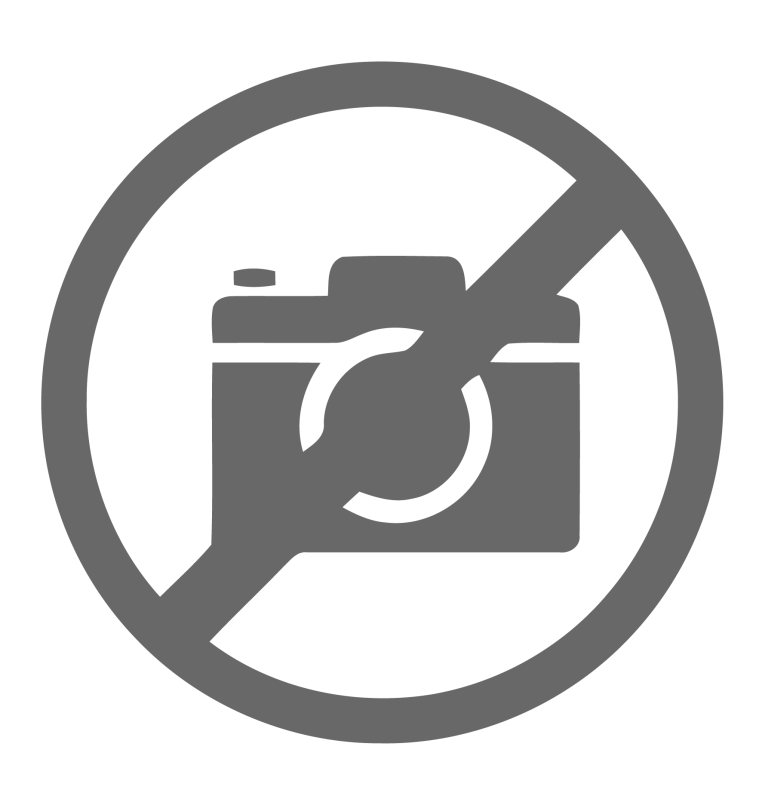
Using AI to optimize ANC performance.
Online optimization of these estimators poses challenges when employing classical signal processing techniques due to a potential lack of reference signals. While estimating Ŝ is relatively straightforward — using music played by the speaker and recorded by the control microphone — estimating Ŝv involves deriving it from Ŝ through some form of identification algorithm.
To address these challenges, neural network-based identification algorithms offer a promising alternative, leveraging the strengths of machine learning to enhance the adaptability and accuracy of noise cancellation systems (Figure 6). For example, they have proven useful in optimizing AF filters to diverse noise conditions [3]. Deep neuralnetworks excel in capturing complex, nonlinear relationships within data. By training these networks on data collected from physical error signals and control inputs and with a substantial training phase, they can predict error signals at virtual locations effectively.
Achievable Performance
Loose-fit models catch up with the performance of occluded ones.
Huawei’s FreeBuds 5 introduced effective noise attenuation in loose-fit designs, setting a new standard for comfort and open-fit functionality. However, Apple’s AirPods 4 advanced this technology further by demonstrating that loose-fit designs can now achieve noise attenuation levels comparable to those of top-performing occluded models.
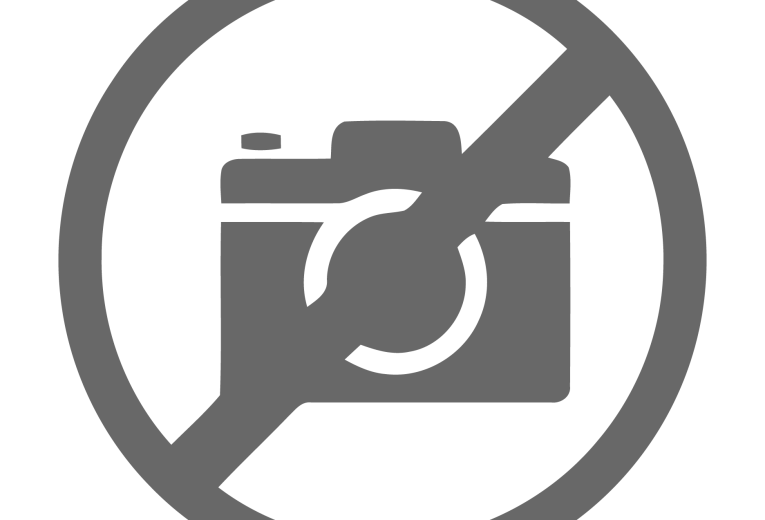
Achievements in OTWS: Cleer Arc III Pro.
In May 2024, Cleer Audio announced the ARC III Pro (Photo 1), a set of open-ear headphones featuring AI-ANC technologies developed by Orosound Labs, a privately held IP provider specializing in AI-driven noise control solutions. The new ARC III Pro generation has received the 2024 Frost & Sullivan certificate [4] for being the first open AI noise-canceling device, setting a new benchmark in the audio industry.
While ANC performance in open-ear designs such as the Cleer ARC III Pro differs from occluded or loose-fit models designed for deep isolation in high-noise environments, significant advancements have been achieved. The long-standing belief that open-ear headphones cannot effectively mitigate ambient noise is being redefined, especially with new technologies such as Orosound Labs’ AI-ANC. With approximately 20dB of noise reduction up to 1kHz, open-ear ANC now achieves about 60% of the attenuation provided by high-performing occluded designs, all while maintaining an open ear canal. This marks a substantial breakthrough for the audio industry, highlighting the potential of open-ear designs to deliver effective noise control.
Attenuation levels aside, it is essential to emphasize the unique approach used in designing ANC for open-ear headphones. While occluded or loose-fit designs are optimized for maximum isolation in high-noise environments, open-ear headphones utilize ANC technology to control ambient noise effectively while preserving the situational awareness and comfort characteristic of open designs (Figure 7). This innovation in ANC technology brings new user benefits, such as enhancing audio immersion without the need for high listening volumes and reducing listening effort — challenges often encountered with traditional OTWS designs.
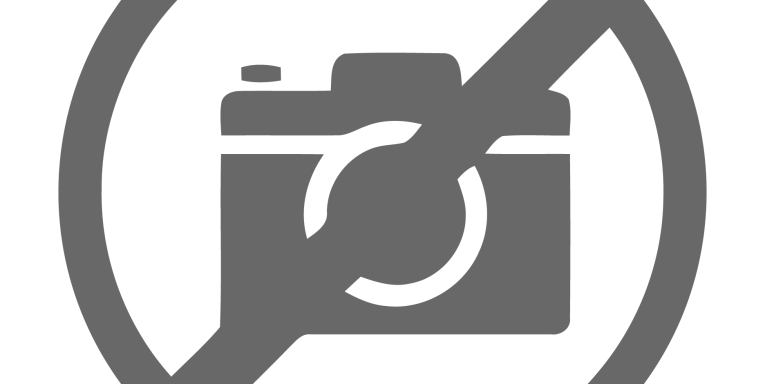
As the hearable market continues to expand, consumer expectations for effective ANC across various form factors are rising. While achieving in-ear-level ANC in both occluded and loose-fit models remains a primary industry goal, open-ear ANC introduces a unique set of challenges. The focus now shifts from complete isolation to providing sound comfort in environments where users need to remain aware of their surroundings.
Advancements in virtual sensing algorithms and the integration of neural networks show promising developments in active noise cancellation, especially for open-ear designs that face unique challenges due to sensor placement constraints. By employing machine learning techniques, particularly deep learning models, these systems can adapt to complex acoustic environments and respond dynamically to factors such as user morphology and device placement.
Next-generation processing will integrate non-acoustic data from sensors, such as head tracking or capacitive sensors, promising to refine noise cancellation even further by accounting for the spatial orientation and fit of devices. Altogether, these innovations lay the groundwork for more versatile and user-responsive noise control systems, enhancing comfort and effectiveness in a wide range of audio environments.
The development of AI-enabled ANC in these innovative form factors is facilitated by a new generation of highly efficient and ultra-low-latency processors tailored for energy-constrained devices and is becoming a technical playground for audio brands. aX
References
[1] D. Moreau, B. Cazzolato, A. Zander, and Cornelis Petersen, “A Review of Virtual Sensing Algorithms for Active Noise Control,” 2008.
[2] M. Pawelczyk, “Adaptive noise control algorithms for active headrest system,” 2004.
[3] B. Wang, D. Shi, Z. Luo, “Transferable Selective Virtual Sensing Active Noise Control Technique Based on Metric Learning,” 2024.
[4] Frost & Sullivan China, www.frostchina.com, 2024.
This article was originally published in audioXpress, February 2025
About the Authors

