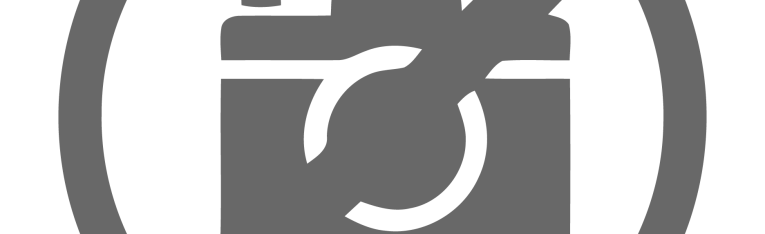
Not all Rub & Buzz faults detected by conventional mechanisms are audible — the masking effects of the human ear and background noises can render some of them inaudible even though they are clearly present when the signal is analyzed. In the quest for higher yields, it’s valid to question whether a speaker with inaudible faults needs to be rejected. This is particularly true for smaller and lower-margin products (e.g., microspeakers used in mobile phones, laptops, and some smart devices). Over the past decade, this desire for lower costs and higher yields has driven the development of accurate perceptual distortion algorithms that reliably identify whether a fault is audible, rather than simply whether it exists.
This article follows the development of production line Rub & Buzz detection, starting with the conventional methods that, although developed 25 years ago, are still valuable and well-used today, and ending with the latest in perceptual analyses. The trade-offs between each are discussed, and the reasons why both conventional (higher-order harmonic) and perceptual distortion measurements provide valuable data points for end-of-line tests are explained. It should be noted that these days, production line Rub & Buzz measurements are equally likely to be used on headphone drivers or microspeakers as they are conventional loudspeakers, and perceptual measurements are rapidly gaining traction in the quest for higher yields of such lower-cost products.
What Causes Rub & Buzz
Rub & Buzz defects are almost exclusively introduced in the manufacturing process. The term “Rub & Buzz” refers to the presence of higher-order harmonics, which create a harsh buzzing sound that often becomes particularly obvious at higher playback levels. Such sounds are created when something abruptly impedes the free movement of the speaker mechanism, for example, loose glue joints, vibrating voice coil lead wires hitting another loudspeaker part (e.g., the cone), or an incorrectly centered and rubbing voice coil.
Naturally a speaker with Rub & Buzz faults sounds bad and should be rejected, but identifying such speakers is just one benefit of measuring this important parameter. Often, Rub & Buzz is caused by improperly configured production line settings, or even a drift in parameters over time. It can therefore affect large volumes of product. Continual monitoring of Rub & Buzz enables trends to be noted and any line adjustments to be made in a timely manner, preventing waste.
Higher Order Harmonic Distortion (HOHD) Measurement—1995
Measurement of higher-order harmonics is often considered a “traditional” Rub & Buzz measurement. This technique, as the name suggests, relies on the analysis of higher-order harmonics — usually the 10th and above. These harmonics contribute most significantly to Rub & Buzz as their distance from the fundamental frequency makes them less masked and more easily detected by the ear. They also tend to fall in the frequency range most sensitive to the ear—around 1kHz to 5kHz. This is not to be confused with the measurement of total harmonic distortion (THD) — a completely different parameter that measures overall speaker harmonic distortion with much of the dominant information coming from the larger second and third harmonics that are excluded from Rub & Buzz detection. Although a valuable metric in itself, THD aligns more to the speaker design rather than manufacturing defects.
Prior to the development of Listen’s higher-order harmonic distortion (HOHD) measurement technique, there were two methods used for measuring it. The “tracking high-pass filter” method, shown in Figure 1, uses a high-pass filter to remove the lower harmonics (usually up to 5th) and sums the remainder to give an overall metric. This method does not distinguish between periodic distortion (Rub & Buzz) and transient distortion (loose particles—see the Sidebar) lumping all the individual harmonics plus transient distortion together. This means that while it gives an overall indication of distortion level, it reveals nothing about the origin of the distortion, a major drawback in terms of understanding manufacturing problems. It is also susceptible to background noise, often resulting in false positives on the production line.

The second method, used in the popular (at the time) Brüel & Kjær 2012 analyzer, sequentially measures individual harmonics using a tracking filter that moves from one harmonic to the next. While this provides accurate and detailed information on each harmonic, it is not fast enough to use on automated production lines.
The launch of SoundCheck in 1995 brought the first production line Rub & Buzz algorithm that is fast, has good immunity to background noise, and provides individual harmonic detail. Using Listen’s proprietary Harmonictrak algorithm, it measures each harmonic discretely and simultaneously, and offers the user complete flexibility in which harmonics are analyzed. The advanced filtering in this algorithm (Figure 2) excludes the noise between the harmonics, which means that in addition to its unprecedented analysis speed, it is also highly immune to background noise—an important characteristic for production line use.
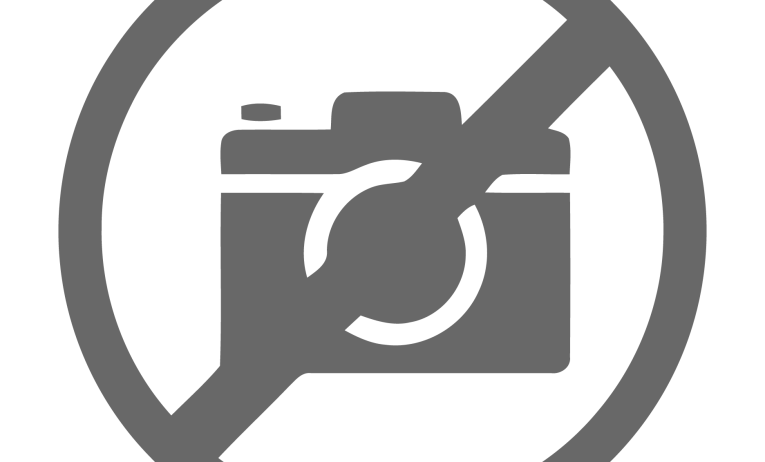
The individual harmonic analysis offered by this technique reveals much about the failure mode, enabling a deeper analysis of the failure mechanism. High even-order harmonics are an indication of an asymmetrical nonlinearity, such as the voice coil not being centered in the magnetic gap. High odd-order harmonics are an indication of a symmetrical nonlinearity, such as clipping or reaching speaker cone and spider excursion limits.
High levels of harmonic distortion in the 10th to 15th order range usually indicate a rubbing voice coil. Distortion at the 50th harmonic and above is frequently caused by voice coil lead wires hitting the cone. Examining the harmonic response of speakers with known faults and carefully selecting the harmonics to analyze can reveal many discrete manufacturing defects. Trends can also be monitored over time, so that production line adjustments can be made if something is drifting out of specification over time. As with other production tests, it uses a stepped sine wave test signal, so the analysis is performed simultaneously with other end-of-line measurements.
Normalized Rub & Buzz Distortion—1996
The next innovation in Rub & Buzz measurement, Normalized Rub & Buzz distortion, came in 1996. I originally devised and proposed this method a few years earlier when working as an applications engineer at Brüel & Kjær, and it was the subject of a 1993 Audio Engineering Society (AES) paper, “How to Graph Distortion Measurements” [1]. SoundCheck was the first test system to include this as an out-of-the box software feature back in 1996, and the method was subsequently endorsed by Dr. Wolfgang Klippel in a 2004 Journal of the Audio Engineering Society (JAES) manuscript [2].
This analysis is very similar to conventional Rub & Buzz distortion—in fact it is probably best described as an enhancement to it. It differs in that the harmonic levels are first compared to the fundamental at their measured frequency, then their ratio is plotted at their excitation frequency. This removes the effect of the frequency response from the distortion — a logical decision since the two are unrelated. This makes it easier to see the peaks in the distortion response, and easier to set limits as the frequency response of each speaker does not need to be accounted for. These are both significant advantages for production line use. Despite this technique being a quarter-century old, it is still used on production lines worldwide, and is just as valid today as when it was first conceived.
Figure 3 demonstrates why normalized Rub & Buzz is more revealing than conventional distortion measurement. The Fundamental (green line) shows a clear roll-off below 400Hz. This causes the conventional Rub & Buzz distortion (red line) to be higher relative to the fundamental in this range, which leads to an overestimation of the distortion level. When the effect of the frequency roll-off is removed, the shape of the curve (blue line) is different, with particular reduction in distortion at low frequencies. This makes the distortion measurement easier to interpret.

It is important to note that this Rub & Buzz detection method, as with conventional higher-order harmonic distortion, does not discern between audible and inaudible faults. This is advantageous in that it will pick up production line inconsistencies even if the fault is inaudible, making it a valuable diagnostic tool [3]. On the downside, speakers with inaudible faults may be unnecessarily rejected, thus reducing yield.
Perceptual Distortion—2011
By the late 2000s the quest for higher yields, particularly for the inexpensive, low-margin miniature speakers widely used in devices (e.g., mobile phones), spurred manufacturers to seek end-of-line tests that would only reject speakers with audible faults. This sparked the development of perceptual distortion algorithms [4]. In 2011, Listen launched the first perceptual Rub & Buzz distortion algorithm, called CLEAR.
This method (Figure 4) applies the psychoacoustic principles outlined in the ITU PEAQ standard for CODEC sound quality evaluation to create an algorithm that replicates human hearing. As with conventional Rub & Buzz measurement, it begins with a stepped sine wave stimulus. Two separate analyses are then carried out on the response signal, which are combined into a numeric perceptual distortion measurement in Phons. In one analysis, the frequency response of the ear is modeled. First, auditory filter bands convert the fast Fourier transform (FFT) spectrum (constant bandwidth) to a Bark scale to replicate ear filtering.
Next, an ear weighting filter compensates for the transfer function of the outer to inner ear, and finally, the internal noise of the ear (noise floor due to blood flow) is added. A frequency spreading function — a simplified mathematical representation of auditory masking curves—is then applied to mimic the psychoacoustic filters of the ear in hearing Rub & Buzz defects. These masking curves change with frequency and level. The fundamental and its masking effects are then removed to give the distortion of the speaker plus noise. This is summed over the frequency range to give the perceptual partial loudness for a single tone of the input signal.
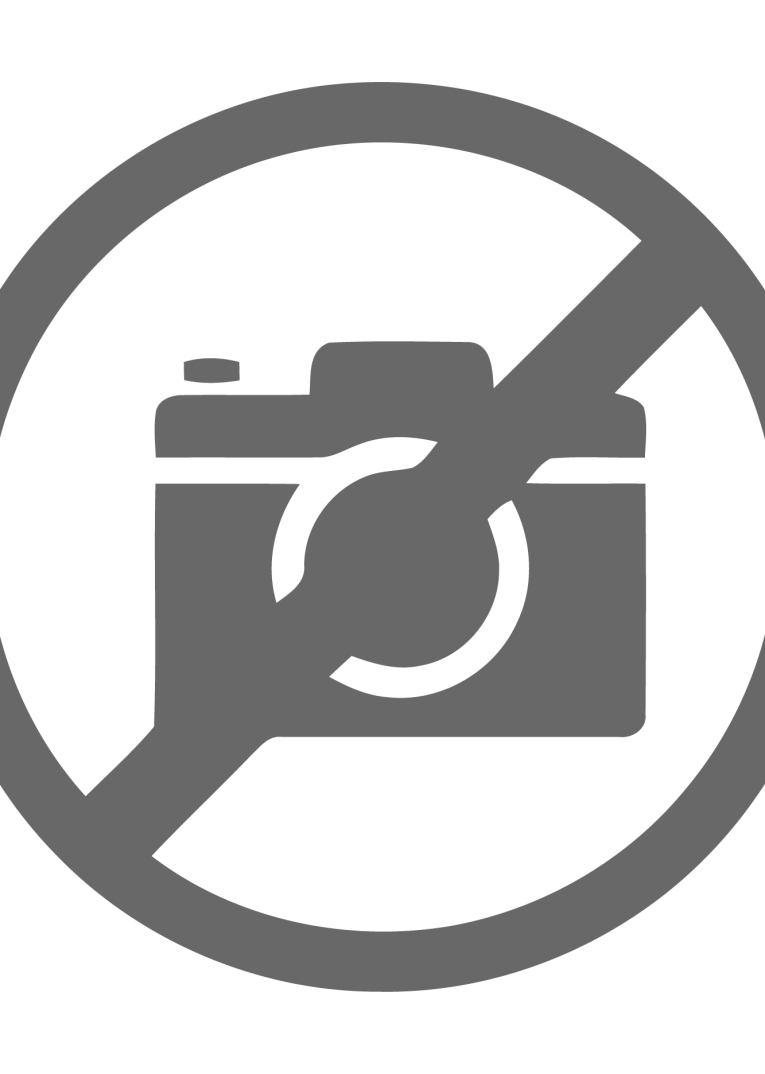
On the other branch of the algorithm, the harmonic structure of the response is quantified using the power cepstrum (a cepstrum is a spectrum of a log spectrum). A strong and extended harmonic structure is a signature of Rub & Buzz.
In the final step of the algorithm, the result of the harmonic analysis is combined with the perceptual distortion to accentuate the Rub & Buzz, and return a harmonically weighted value for Rub & Buzz loudness in Phons [5, 6, 7].
This algorithm truly was a first in this industry, offering excellent correlation to human hearing. Although it was well received, its sensitivity to background noise made the repeatability inconsistent, and consequently made it challenging to set limits. This hampered its adoption in noisy production environments.
Overcoming these issues has not been an easy task; the fact that it was a good 10 years before any other test manufacturer launched a perceptual Rub & Buzz metric is testament to the magnitude of this challenge. Indeed, more than 10 years later, some of the other recently launched algorithms suffer from the exact same repeatability issues and dependencies as Listen’s original CLEAR algorithm.
Enhanced Perceptual Rub & Buzz (ePRB) Distortion—2021
Enhanced Perceptual Rub & Buzz (ePRB) Distortion, Listen’s newest perceptual Rub & Buzz algorithm has just been released with SoundCheck 20. This new algorithm, the product of several years of original research, builds on the sound psychoacoustic principles of the original CLEAR algorithm, but features two significant enhancements to improve performance (Figure 5). First, a new perceptual filtering algorithm significantly improves correlation to human hearing. Second, a novel noise-reduction algorithm offers significantly improved performance in noisy environments. This makes limit setting easier, and increases repeatability.

As with the original perceptual Rub & Buzz algorithm, this test method also uses a stepped sine sweep stimulus, enabling it to be measured simultaneously with other end-of-line quality metrics with no increase in test time. The underlying principles of this algorithm are well documented above and in several AES papers [5, 6, 7], so here the focus is the results of the recent enhancements.
Figure 6 compares the same speaker using both the original perceptual Rub & Buzz metric and the new enhanced version. The new algorithm shows much greater distortion in the mid-frequency range. This reflects the enhancements to the perceptual algorithm that make it more accurately model the behavior of the human ear in the highly sensitive mid-range frequencies. Hundreds of speakers were subjectively and objectively tested during the design of this algorithm, and the correlation with subjective opinion was significantly higher than with the prior version.
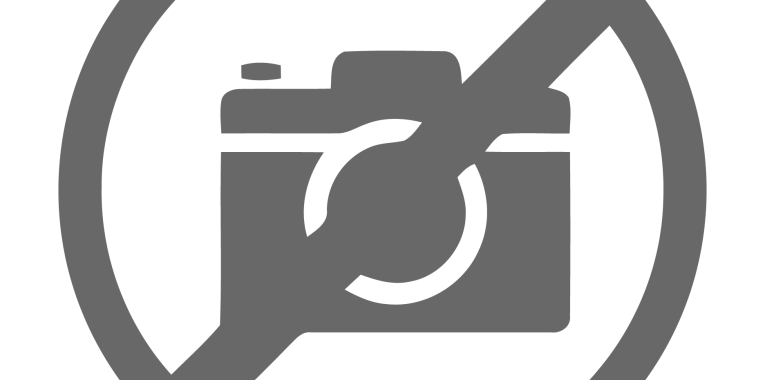
Next, we compare good, bad, and borderline speakers using Listen’s original 2011 algorithm, Listen’ new 2022 algorithm, and another recently launched perceptual Rub & Buzz algorithm. Three speakers of the same type—a typical small loudspeaker—were analyzed.
One speaker is good, one has high levels of Rub & Buzz distortion, and the third exhibits some distortion, but it is not as audible as in the speaker with high distortion. These three particular devices were chosen from a much larger batch as examples of good, mildly distorted, and severely distorted output in order to clearly show contrast in the results. The three speakers were subjectively assessed by a panel of engineers experienced with listening to distortion artifacts, and the results are shown in Table 1.
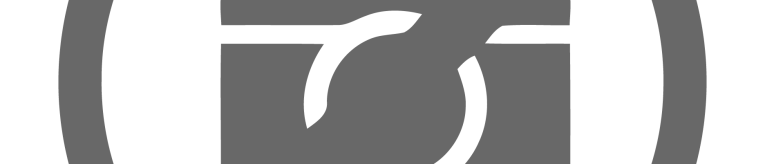
Figure 7 shows the results of these three speakers tested 10 times each in an ordinary room, and measured with the three different algorithms outlined in Figure 7 — Listen’s original Perceptual Rub & Buzz (a), the new enhanced Perceptual Rub & Buzz (b), and the other recently launched algorithm “Other Perceptual Algorithm” (c).
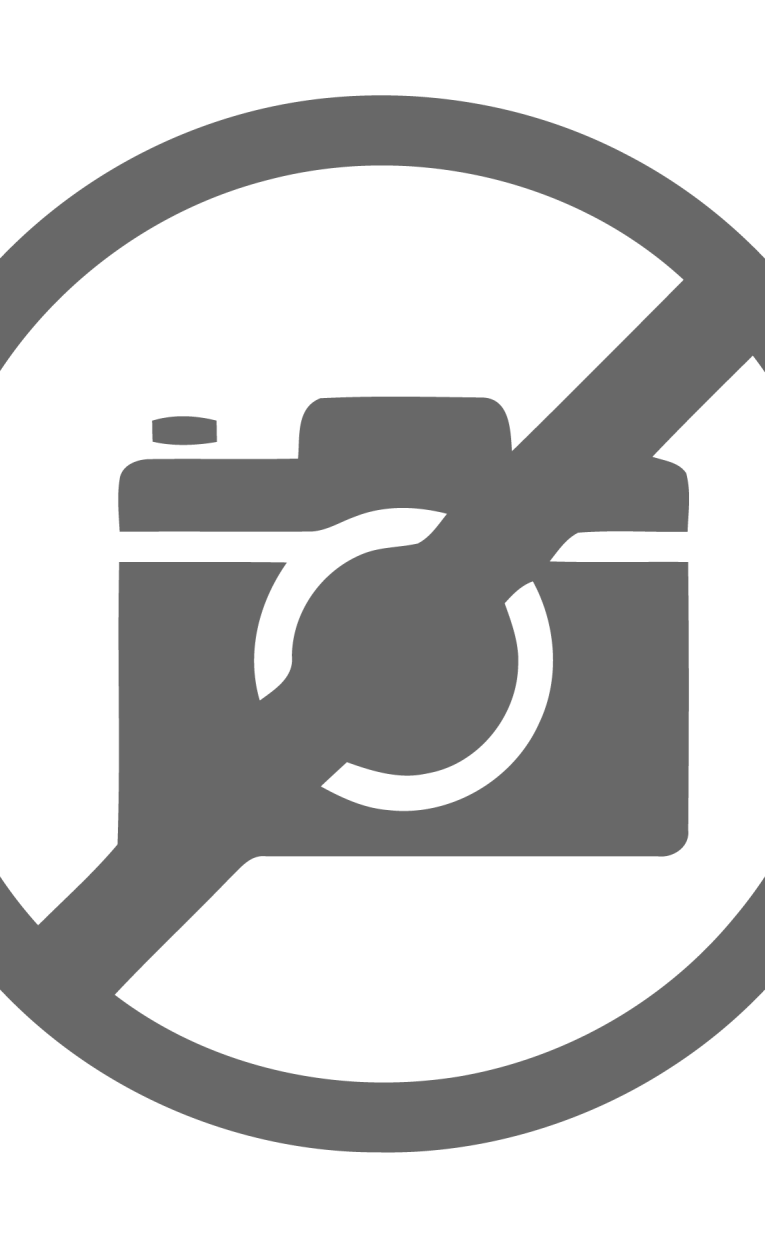
In Figure 7a, the original (2011) perceptual algorithm visually indicates the distortion faults, clearly showing correlation to subjective listening results. The bad speaker shows the highest level of audible distortion, the good speaker has minimal measured distortion, and the borderline speaker is somewhere in the middle. However, when the speakers were measured 10 times, small variations in the results are observed. This is primarily due to background noise, and in a production environment where there is only one test sweep on each device, this variation makes limit-setting challenging.
Figure 7b shows Listen’s new (2022) ePRB algorithm, also with the exact same 10 measurements for each speaker. It is immediately apparent that the results are more consistent, as is expected with less sensitivity to noise. This improved repeatability makes it considerably more reliable than the original algorithm and easier to set limits, therefore making it more suitable for production line use. Additionally, the enhancements to the algorithm reveal increased detail in the ear’s highly sensitive 500Hz to 2kHz range, which more accurately mirrors human perception.
Figure 7c shows another recently launched perceptual Rub & Buzz algorithm. This demonstrates similar repeatability issues to SoundCheck’s 2011 algorithm, and, interestingly, it measures greater audible distortion at low frequencies for the speaker that was subjectively assessed as borderline than the one subjectively assessed as bad.
These results are validated by examining the harmonic distortion using “conventional” FFT analysis (Figure 8), as well as correlating them to listener experience. To simplify both the listening experience (it’s very hard for even a trained listener to hear distortion in a fast sweep) and the harmonic spectrum, a fixed frequency of 256Hz was used to evaluate the same three speakers. This frequency was chosen as it reflected the frequency at which both bad speakers exhibit a peak in perceptual distortion measurement and therefore would be the easiest to hear.

The distortion spectra clearly shows minimal high-order harmonic distortion for the good loudspeaker (green line), which correlates well with the perceptual measurement, as one would expect. Both the bad and borderline speaker show elevated high-order harmonic distortion, and again, in line with Listen’s perceptual algorithms, the bad one (red) clearly has more distortion than the borderline one throughout the spectrum. At no point does the borderline (orange) speaker exhibit more distortion. As one might expect, the perceptual distortion algorithm amplifies the relative magnitude distortion in the highly sensitive part of the ear’s range.
It is interesting that the “other” perceptual algorithm shows little difference in the maximum distortion audibility of the two speakers that exhibit Rub & Buzz, although human listeners perceived a significant difference in distortion level. Furthermore, at the 256Hz frequency measured above, it actually showed greater distortion with the borderline speaker (orange line) than the bad speaker (red line), which does not correlate with either the HOHD measurement or the listener experience.
Distortion Parameters for Assessing Production Line Defects
Generally speaking, there are three valuable distortion parameters that should form part of your end-of-line test for assessment of manufacturing defects: individual harmonics distortion, loose particles, and perceptual Rub & Buzz. Each serves a particular purpose.
Normalized Rub & Buzz (or HOHD) will identify any fault on your production line that is leading to a rubbing or buzzing device, whether you can hear it or not. Trends can be monitored over time to catch any production line drift before speakers start being rejected. An analysis of the specific harmonic that is causing the device to fail can provide valuable insight into what the specific problem is, accelerating production line troubleshooting and enabling problem areas to be addressed. Although standard discrete higher-order distortion measurement will also suffice, the normalized version is more accurate and more revealing. It also makes it considerably easier to see the peaks in response and set limits — important characteristics for production line use.
Loose particle detection rejects speakers with transient faults. This tends to be caused by the ingress of foreign particles into the speaker during manufacture and tends to occur on a more random basis. Nevertheless it still sounds unpleasant to the human ear and speakers with this defect in the audible range should always be rejected.
Perceptual Rub & Buzz is an indicator of whether the consumer will be able to hear the fault. In some situations, particularly with lower cost devices, a knowledge of whether the distortion is audible may affect the decision on whether to pass or fail it. In other words, if a speaker’s Rub & Buzz defect is not audible, in many cases it may be acceptable to ship it, since the consumer will never hear it. This reduces manufacturing waste, and therefore increases yield/profitability for the line.
All three measurements use a stepped sine stimulus. This means that they can be measured and analyzed simultaneously (along with other production line metrics such as frequency response, THD, polarity, impedance including Thiele-Small parameters and more) in a single sweep (Figure 9). Typically this takes as little as one second, making measurement of all of these parameters fast and easy on today’s high-speed production lines. Whatever other end-of-line measurements are made, these three will enable you to accurately identify common manufacturing defects and remove the affected speakers.
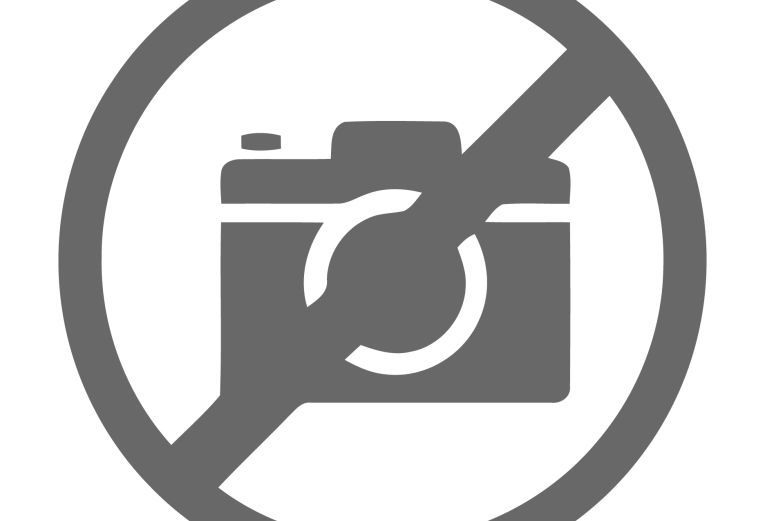
The Future of Production Line Distortion Measurements
Perceptual measurements are the “holy grail” of production electroacoustic measurements because of their potential to increase the yield of high-quality products in a cost-driven marketplace. This will likely result in many innovations and algorithms in the next few years that better correlate manufacturing defects to human auditory perception, and will likely include some overall psychoacoustic and distortion metrics. aX
Loose Particles
It is important that Rub and Buzz measurements, perceptual or otherwise, are not confused with Loose Particles or other randomly occurring transient defects. Like Rub & Buzz, loose particles cause an unpleasant clicking or rattling sound within the speaker, and affected speakers should be similarly rejected. However the failure modes that cause loose particle defects, and the method used to detect them, are completely different. Loose particles are caused when foreign particles such as glue or magnet fragments become trapped in the gap behind the diaphragm or dust cap during the manufacturing process.
The random clicking, popping or rattling noises made by these trapped particles cause transient, rather than periodic distortion. This is identified by time-envelope analysis of the waveform response in the time domain (Figure 10). Detection thresholds are set for the magnitude, duration, and number of transients to quantify their level and number over time. Limits and filters are applied to separate device transients from background noise. Fine tuning with these tools customizes the algorithm for your product and manufacturing environment [8, 9].

[1] S. Temme, “How to Graph Distortion Measurements,” presented at the Audio Engineering Society (AES) 94th Convention, March 1993.
[2] W. Klippel, “Measurement and Amplification of Equivalent Input Distortion,” Journal of the Audio Engineering Society (JAES), Volume 52, Issue 9, pp. 931–947, September 2004.
[3] D. Foley, Dr. R. Celmer, B. Sachwald, J. Anthony, T. Pagliaro, and S. Thompson, “Higher Order Harmonic Signature Analysis for Loudspeaker Defect Detection,” presented at the 117th Audio Engineering Society (AES) Convention, October 2004.
[4] S. Temme, P. Brunet, and D.B. Keele Jr., “Practical Measurement of Loudspeaker Distortion Using a Simplified Auditory Perceptual Model,” Presented at the 127th Audio Engineering Society (AES) Convention, October 2009.
[5] S. Temme, P. Brunet, and B. Fallon, “Practical Implementation of Perceptual Rub & Buzz Distortion and Experimental Results” Presented at the 129th Audio Engineering Society (AES) Convention, November 2010.
[6] S. Temme, P. Brunet, and P. Qarabaqi, “Measurement of Harmonic Distortion Audibility Using a Simplified Psychoacoustic Model,” presented at the 133rd Audio Engineering Society (AES) Convention, October 2012.
[7] S. Temme & P. Brunet, “Measurement of Harmonic Distortion Audibility Using a Simplified Psychoacoustic Model – Updated,” presented at the 51st Audio Engineering Society (AES) International Conference, August 2013.
[8] P. Brunet, E. Chakroff, and S. Temme, “Loose Particle Detection in Loudspeakers,” presented at the 115th Audio Engineering Society (AES) Convention, October 2003.
[9] P. Brunet and S. Temme, “Enhancements for Loose Particle Detection in Loudspeakers,” presented at the 116th Audio Engineering Society (AES) Convention, May 2004.
Resource
Listen, Inc., www.listeninc.com
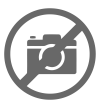
Steve Temme is founder and President of Listen, Inc., manufacturer of the SoundCheck audio test system. Steve founded the company in 1995, and for the past 25 years the company has remained on the cutting edge of research in audio measurement, regularly introducing new measurement techniques, algorithms, and hardware to enable testing of all kinds of devices ranging from basic transducers to complex audio systems. In recent years, Listen’s product development focus has been on creating the algorithms, interfaces and test methods to measure today’s many and varied wireless and cloud-communicating devices. Prior to founding Listen, Steve worked for many years as an acoustic test and measurement applications engineer at Brüel & Kjær, and also as a loudspeaker design engineer at Apogee Acoustics. He holds a BSME from Tufts University, has authored numerous papers on acoustic testing, and has lectured extensively throughout the world.
This article was originally published in audioXpress, April 2022.