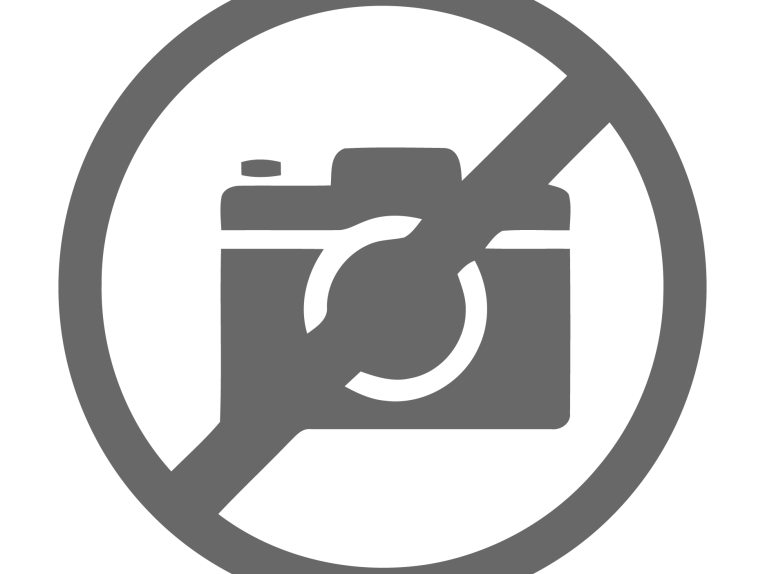
Environmental noise in daily life is increasing, while users still expect to use their audio and communication equipment in all daily life situations. Smartphones providing speech enhancement of the receive-side signal and headphones providing noise cancellation functionality are a logical technical remedy for this issue and are becoming increasingly popular.
Besides reducing ambient noise, these devices include processing technologies that may amplify or attenuate speech signals but also may degrade the speech signal quality. When speech is part of the environmental noise, attenuation may be intentional. However, speech often is the desired signal and in consequence should be enhanced rather than attenuated or degraded.
Although a lot of effort has been spent on the development of objective, perceptual-based methods [1], [2] until recently no method was available for determining the speech intelligibility or the Listening Effort at the receive side of telecommunication systems. So far, it has been impossible to predict the performance increase by any type of adaptive voice enhancement processing objectively and adequately.
To overcome this situation, some years ago HEAD acoustics started to develop a perceptual-based method targeting the objective prediction of Listening Effort for a variety of technical systems:
• Active Noise Cancellation (ANC)
• Near-end voice enhancement, including all types of signal processing such as companding, expanding, adaptive level control, adaptive frequency response shaping bandwidth-extension
• Voice enhancement used in real-time systems/ announcement systems
The application areas for such a method are:
• Smartphones and other smart devices (smart home)
• Headphones and headsets especially with ANC technologies
• Hands-free, conference and car hands-free
• In-Car Communication (ICC)
This work results in a new objective method for determining Listening Effort called “ABLE” (Assessment of Binaural Listening Effort), which was recently standardized in ETSI TS 103 558 [7] and which is available as a standardized option for the voice and audio quality measurement and analysis software ACQUA. This article introduces the basics of the underlying auditory tests, a description of the prediction model and application examples.

Finding the Right Data Set
As a basis of any objective prediction model, listening examples are required to train and validate the model. These listening examples need to cover the entire quality range of devices for which the model is to be developed. Furthermore, these listening examples need to include all impairments which may arise from signal processing and which are relevant for the user’s subjective impression.
When developing an objective test method, in consequence different applications, ambient noises, devices, implementations and possible speech processing algorithms need to be taken into account. In order to predict all these scenarios reliably, numerous noisy speech samples have to be available. To generate these types of listening examples, advanced signal processing techniques for simulation as well as a big variety of devices should be available. In addition, a variety of realistic noise scenarios where these devices are used in, need to be available.
A typical setup for generating such listening examples, which also is used for testing real devices, is shown in Figure 1. Here, the example of testing an ANC headset in conjunction with a Head-and-Torso Simulator (HATS) is illustrated. Pre-recorded background noise is played back in a laboratory using a standardized sound-field generation system (ETSI TS 103 224 [3]). This setup reproduces various background noises representative for the typical environmental noise situations with a high degree of accuracy around the device under test (DUT).
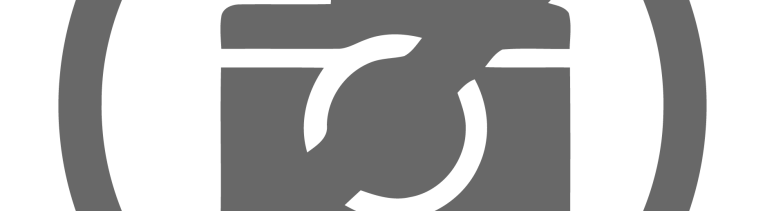
Auditory (Subjective) Test Databases
When evaluating Listening Effort subjects provide a self-assessment on a five-point categorical scale, similar to well-known speech quality testing methods. For evaluation of Listening Effort, the scale shown in Table 1 is used.
The scale and the corresponding attributes are taken from Recommendation ITU-T P.800 [4]. Besides the aforementioned benefits of Listening Effort testing, recent studies (e.g., [5, 6]) indicate that speech enhancement benefits can be evaluated in a wider range of signal-to-noise ratios (SNRs) without reaching positive or negative saturation observed in intelligibility tests.
Auditory tests can be conducted in groups in parallel. A set of test conditions are combined in a test database. Each test database contains a set of speech samples processed with different devices and/or algorithms ideally covering the complete range of Listening Effort and a set of reference conditions.
Mapping of different test databases to each other is realized by mapping to the reference conditions. The purpose of auditory tests is twofold:
• So-called training databases are used to develop and train the objective model for best performance.
• So-called validation databases are needed after model development is completed. The auditory test results of these databases were never seen before by the model. They provide information about the robustness and validity (generalization) of the objective model.
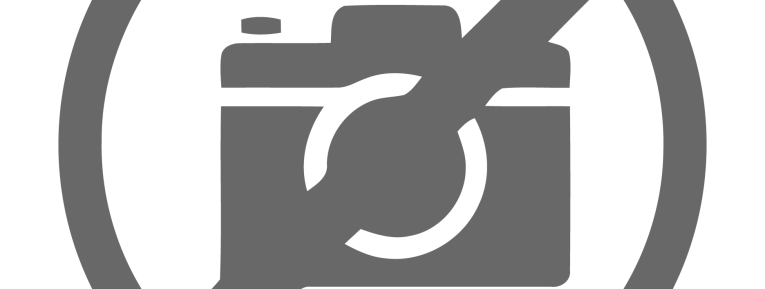
The Instrumental Model ABLE
ABLE, the instrumental model for Listening Effort was recently standardized in ETSI TS 103 558 [7]. A detailed description about the model can be found in this standard. Figure 2 provides an overview of the prediction algorithm, which consists of different stages. ABLE is a binaural model and in general the input to the model are binaurally recorded scenarios.
The binaural input signals to the model are dL(k) and dR(k). The reference signal r(k) is the clean speech signal, which is inserted in the DUT. After pre-processing, a transformation into the time-frequency domain based on a hearing model is applied (time index i, frequency bin j). These signal representations are denoted with capital letters D(egraded), N(oise), P(rocessed) and R(eference). The processing is performed for the left and right ear separately.
Pre-Processing
The purpose of the pre-processing stage is the temporal and level alignment of all input signals. The delay between degraded signals d(k) and the reference r(k) is calculated by a cross-correlation analysis for both ears. The reference signal r(k) is scaled to a fixed active speech level (ASL) of 79 dBSPL (rOpt(k)), which is assumed to be optimal regarding Listening Effort [8, 9]. The transfer functions H(f) between degraded and reference signal are calculated by the H1 methodology (crosspower spectral density) in order to exclude non-correlated noise components.
Hearing Model Calculation
An aurally adequate transformation is performed in the next step using the HEAD acoustics hearing model [10,11]. This transformation is applied to each of the binaurally recorded signals as well as to the clean speech reference signal. The transformation includes an auditory filter bank representation of the signal and a hearing-adequate envelope. This results in a frame resolution of about 8 ms. The initial frequency bandwidth Δf(f0) is set to 70 Hz. In total, 27 hearing-adequate bands up to 20 kHz are used. This time-frequency representations result in the hearing model spectra vs time DL(i, j), DR(I,j) and ROpt(i, j) (see Figure 2).

Separation of Speech and Noise
For the Listening Effort calculation, the degraded spectra D(i, j) are separated into sections with (processed) speech (P(i, j)) and sections representing the noise (N(i, j)) component. For this purpose, a (pseudo-)Wiener filter is used here for the determination of P(i, j). Further details can be found in [7].
For the determination of N˜ (i, j), first a soft mask M(i, j) of active/inactive time-frequency bins is determined with RComp(i, j). The speech part classification is performed for each frequency band. For silent and paused frames, a mask value of 1.0 is set, for high activity of speech to 0.0. Weights for medium, low and uncertain activity frames are calculated by linear interpolation. An example of this threshold-based method for one single frequency band is provided in Figure 3.
The degraded spectra are multiplied by the masks and an estimate N˜ (i, j) of the noise signals per frequency band are calculated via deconvolution. N˜ (i, j) is then used to estimate P(i,j) with the aforementioned Wiener filter and the estimated noise spectra are used as the representation of the noise itself:
N(i, j) ≈ N˜ (i, j).
Binaural Processing
Besides its ability to identify the sound source direction, the human ear is capable to improve the SNR when listening binaurally as compared to monaural listening. This is modeled in the binaural processing block of the prediction model.
The spectral components for left and right ears are combined by the short-term equalization/cancellation methodology as described in [12], which is an extension to the well-known model of Durlach [13]. This block requires the availability of the isolated speech and noise (masking) components. At the output of this block combined and enhanced hearing model spectra vs time are provided.
Metric Calculation
A variety of metrics can be calculated based on the binaural spectra. For ABLE, level-based and correlation-based metrics were chosen. For the level-based metrics, the ASL is used for those segments, which were classified as active speech based on the frame classification of the preprocessing. In addition, an A-weighted noise level is calculated from the noise spectrum.
The correlation-based metrics are derived from a spectral cross-correlation, which is carried out in four different ways. First, the threshold of hearing is applied [14] to the spectra. After this step, the nonlinear loudness transformation according to [10, 11] is applied to all spectra. Each band is subdivided into sub-frames of 320 ms (N = 40 frames, only active indices) with 50 % overlap. These are aggregated to an overall metric. More information can be found in [7].
MOS Calculation
From the metric block, various single values are available and combined to an instrumental single Listening Effort score. Machine learning procedures are used here for the derivation of the instrumental Mean Opinion Score (MOS). Due to the limited amount of training databases available until now, multivariate adaptive regression splines (MARS) according to [15] are used in the current model in order to avoid overfitting.
Parameters and coefficients of the regression are derived from data of similar studies related to the auditory assessment of Listening Effort [16, 17].
Applications of ABLE
As outlined before, the objective model ABLE can be used for various devices and applications. Two rather different application examples are chosen to demonstrate the capabilities of ABLE – ANC headsets and In-Car Communication (ICC) systems.
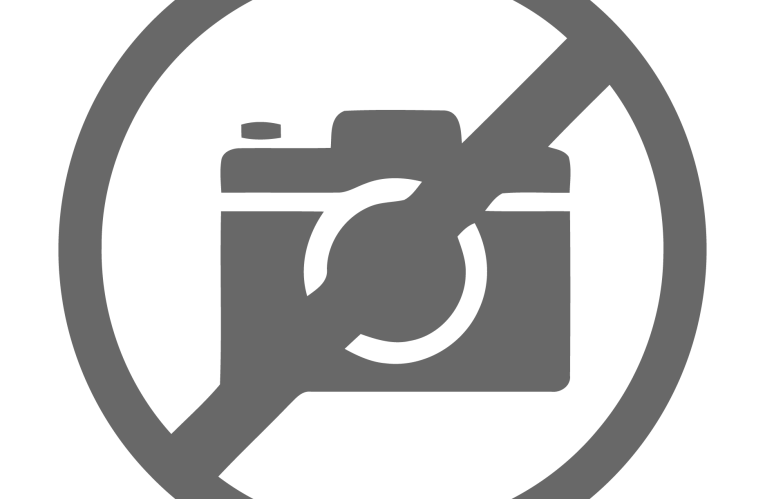
ANC Headsets
Besides active noise cancellation, ANC headsets are often used for communication with ANC active; many devices also offer a so-called talk-through function, which allows passing the speech from a person or from an announcement system. A typical use case is the aircraft, where ANC functionality is in general highly desired, but communication with the service staff or listening to the announcements before/during takeoff and landing is required without removing the ANC headset.
To illustrate the capabilities of ABLE, four commercially available ANC headsets (here denoted as DUT A to D) were evaluated with the measurement using the setup described in the introduction (see Figure 1). DUT A, C, and D represent circumaural headsets, while DUT B is an in-ear device.
Two noise types were used for the noise field simulation according to [3]:
• Train compartment: This scenario was recorded in particular for this evaluation. On average, the noise level is ≈ 64 dBSPL(A), measured close to the ears.
• Airplane: The aircraft noise is taken from the noise database of [3]. On average, the noise level is ≈ 80 dBSPL(A), measured close to the ears.
When evaluating the Listening Effort for the different use cases, speech signals are inserted in various ways. The type and calibration of speech playback depends on the specific use case, two of them are described in the following clauses. The background noise playback is always presented synchronously with the speech signals, synchronized with the start of the measurement. So each device is exposed to exactly the same mixture of speech and noise.
The speech signal used for all evaluations is a speech sequence consisting of 16 American English sentences according to Annex C of ETSI TS 103 281 [2]. The binaural signals are captured at the drum reference point (DRP), a diffuse-field (DF) correction is applied (see [18]).
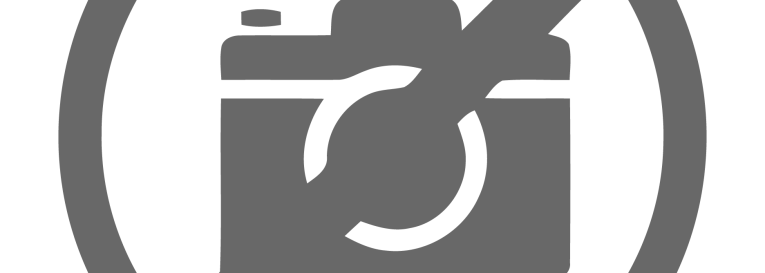
Communication Mode
A typical application of an ANC device is the usage as a headset (i.e., the device) is connected to a mobile phone or computer Figure 4: Test setup of ANC headphones in communication mode for telecommunication purposes. After connecting, the headset establishes a voice call and the speech signal is inserted into the downlink (receive direction). The measurement setup is shown in Figure 4. The headsets investigated were connected to a Bluetooth reference gateway. The connection was established with hands-free profile in wideband mode, which allows an audio bandwidth up to 8 kHz. The active speech level (according to ITU-T P.56 [19]), measured at the ear under silent conditions was adjusted to a range of 73 to 79 dBSPL per ear, which is considered as an optimum level regarding Listening Effort and quality [8], [9]. This level was adjusted individually for each device. For all devices, ANC functionality was enabled and set to maximum cancellation operation mode where possible.
The results for the different headphones in communication mode for the different noises are shown in Table 2. As expected, the Listening Effort decreases significantly with increasing noise levels, which can be seen when comparing results between train compartment and airplane noise scenarios.
The speech level Sact values remain stable within a range of ~74-80 dBSPL, while the noise cancellation performance and impact on MOSLE differ, depending on scenario and device. The remaining noise level between the devices varies up to 17 dB. Even though DUT C provides quite low speech levels in both noise scenarios, this device outperforms the others with respect to residual noise level and MOSLE = 2.8 is assessed for the most demanding noise type “airplane.” The lowest MOSLE is measured with device A. The rank order in performance also depends on the type of noise. While for airplane noise headset C outperforms the other devices, headset B performs best with train compartment noise. In general, it would be expected that speech playback via headphone would result in even better values for MOSLE. However, many devices include amplification of the audio signal in the low-frequency domain, which relatively reduces high-frequency components important for speech intelligibility.
Further impairments may be due to non-optimum frequency response shaping, bandwidth limitations and speech coding which degrades the quality of the transmitted signal.
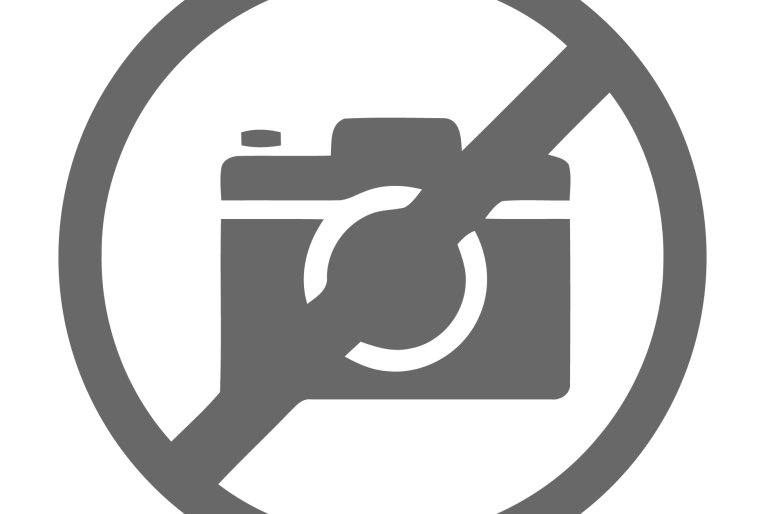
Talk-Through Mode
Speech signals originated from the surroundings (e.g., another talker or public address systems) are not easy to identify as wanted signals and may be attenuated in the same way as the environmental noise. To overcome this challenge, many devices employ a so-called talk-through (TT) functionality, which is evaluated more in detail.
To evaluate the talk-through performance of the devices, the talker can be simulated by a second HATS positioned at a distance of d = 50 cm to the left of the listener as shown in Figure 5. The artificial mouth of the second artificial head is equalized up to 20 kHz, providing most natural presentation of the second talker’s voice. The speech signal level is -4.7 dBPa in silence, in noisy scenarios the speech signal level is increased considering the Lombard Effect (humans talk louder in noise scenarios) as described in [20]. The ANC functionality is enabled and set to maximum cancellation operation mode for all devices. The corresponding level settings are shown in Table 3.

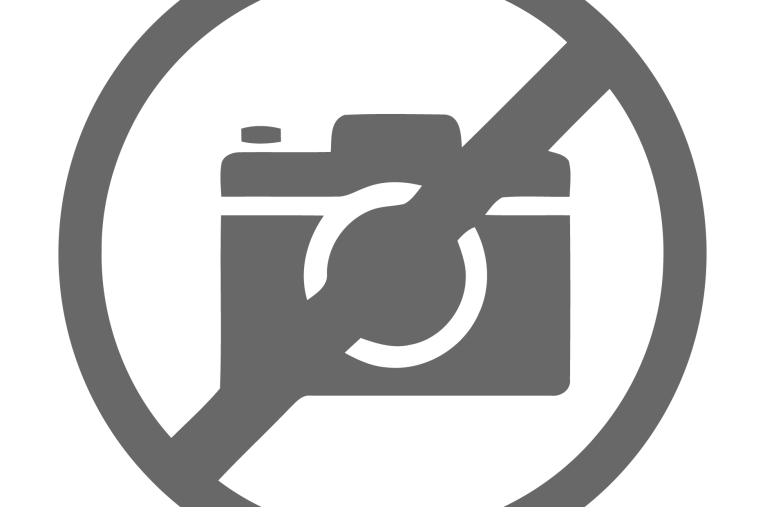

Besides the communication to a human, the talk-through mode is useful for listening to announcement systems (e.g., in an airplane). For this simulation, an equalized loudspeaker is positioned at a distance of 50 cm a height h = 50 cm above the horizontal plane of the artificial head and moved 45° in front of the listener (see Figure 6). The loudspeaker output is calibrated to obtain a typical and reasonable level and SNR at the listener’s ears (without wearing a device) for such scenarios. The corresponding level settings are shown in Table 4.


Table 5 and Table 6 show the prediction results for the talk-through mode with a second talker and a PA-simulation (the loudspeaker scenario) for both noise scenarios across the four headsets. In the talk-through scenario with a second talker (Table 5), the speech level Sact decreases compared to the communication mode (in some cases more than 10 dB), due to active and passive attenuation of any sound field outside the headset. For the second talker scenario, the noise cancellation performance remains comparable across devices and noise types. The speech attenuation is higher than in communication mode, in some cases up to 9 dB (DUT D for train compartment).
Obviously, the noise cancellation performance is reduced compared to communication mode, where speech is simultaneously played back. Nevertheless, the MOSLE is comparable. Listening Effort is less sensitive against the speech level itself. Especially for low SNR ranges, the noise level reduction seems more important.
Table 6 shows the prediction results for the talk-through mode with an additional loudspeaker for the two noise scenarios. In this setup, a dedicated talk-through mode was activated. The noise cancellation performance decreases although the speech level increases compared to the previous talk-through mode.
The Listening Effort does not seem to benefit from this increase; results for MOSLE are consistently lower. The concurrent gain of noise level is predominating here. It becomes clear that the dominating factors for the assessment of Listening Effort are the (residual) noise and the (mere) speech level. However, it is not sufficient considering just the SNR for the Listening Effort. It seems more important to investigate at which speech/noise level a certain SNR is obtained.
This can be seen for the talk-through scenarios, where wide ranges of speech and noise levels are covered.

In-Car Communication (ICC)
Another application area for ABLE is evaluation of ICC systems. ICC systems are used to enhance the communication between passengers in a car. Conversation between passengers may be impaired due to the driving noise present and the high speech attenuation, which is caused by e.g., an acoustically high-quality treated car or higher distances between talker and listener. The bigger the car, the bigger the challenge.
The performance of ICC systems is measured by a variety of parameters, which can be found in the newly released Recommendation ITU-T P.1150 [21]. One very important question is how ICC systems can improve the Listening Effort in such situations. ABLE is ideally suited for this application. Based on a variety of subjective studies, a requirement for the enhancement provided by ICC systems was developed (see Figure 4).
From Figure 7, it can be seen that the required enhancement provided by ICC systems depends on the measured performance without ICC. The explanation for this requirement is rather easy. For lower Listening Effort values, the noise level in the car is generally high. However, the speech signal amplification introduced by the ICC system is limited. Even in noise, too high speech levels decrease the Listening Effort and even may damage the user’s ear.
On the other hand, with low noises in a car there is often not much enhancement needed. Under quiet conditions, Listening Effort is already sufficiently good in smaller cars. In bigger cars and cabins with three rows, Listening Effort may already be quite poor, just due to the insufficient level of the direct speech. Therefore, any reinforcement introduced by the ICC system would well improve the situation. This is the best operation range of ICC systems.
Figure 8 provides some examples how these requirements are applied on several ICC systems under different driving/noise conditions. It can be seen that it is technically possible to achieve such a degree of improvements. ABLE is a suitable methodology for predicting the Listening Effort improvement according to ITU-T P.1150 [21], but can also be used for optimization of ICC systems as well.
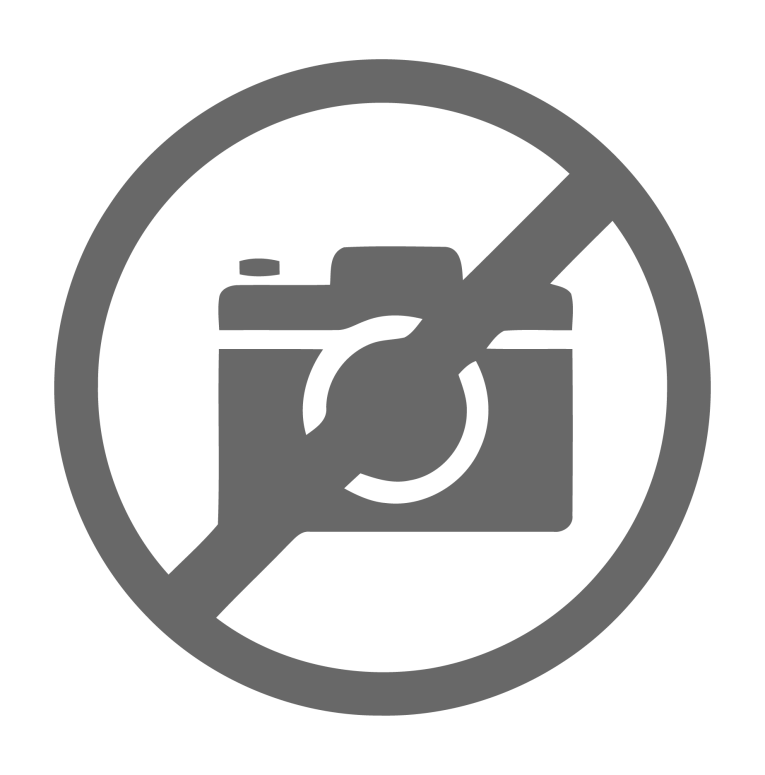
Conclusions and Outlook
With ABLE, a new perceptual-based method is available allowing the objective evaluation of Listening Effort for a variety of devices, for a variety of applications in various situations. Besides the qualification of devices, ABLE is perfectly suited for the performance optimization of (tele-) communication devices. Work for further enhancement and validation of this methodology is ongoing in ETSI Technical Committee STQ.
ABLE is commercially available. In conjunction with background noise and room simulation techniques [3], a laboratory setup including an artificial head, turntable (depending on application) or car environment as well as a fully automated test system, reliable measurements can be conducted in a laboratory environment. This setup enables developers and test labs to efficiently and reproducibly optimize and qualify numerous types of devices. aX
This article was originally published in audioXpress, April 2020.
About the Authors
Hans W. Gierlich started his professional career in 1983 at the Institute for Communication Engineering at RWTH, Aachen. In February 1988, he received a Ph.D. in electrical engineering. In 1989, Gierlich joined HEAD acoustics GmbH in Aachen as vice president. Since 1999, he is head of the HEAD acoustics Telecom Division and in 2014, he was appointed to the board of directors. Gierlich is mainly involved in acoustics, speech signal processing and its perceptual effects, QOS and QOE topics, measurement technology and speech transmission quality. He is active in various standardization bodies such as ITU-T, 3GPP, GCF, IEEE, TIA, CTIA, DKE, and VDA and chairman of the ETSI Technical Committee for “Speech and Multimedia Transmission Quality”.
Jan Reimes started his academic studies of electrical engineering at RWTH Aachen, Germany in 2000. In 2006, he received his diploma degree in information and communication technology with final thesis at the Institute of Communication Systems. From 2006 to 2015, he was employed at HEAD acoustics GmbH for the Telecom development department. In 2016, he joined the newly founded department “Telecom Research & Standardization”. His fields of interest and research are in measurement technology and perceptual based models. He is active in a variety of standardization bodies such as ITU-T, ETSI and 3GPP.
About HEAD acoustics
HEAD acoustics GmbH is one of the world’s leading companies for integrated acoustic solutions as well as sound and vibration analysis. In the telecom sector, the company enjoys global recognition due to the expertise and pioneering role in the development of hardware and software for the measurement, analysis and optimization of voice and audio quality as well as customer-specific solutions and services. HEAD acoustics’ range of services covers sound engineering for technical products, investigation of environmental noise, speech quality engineering as well as consulting, training and support. The medium-sized company from Herzogenrath near Aachen has subsidiaries in China, France, Italy, Japan, South Korea, the UK and the USA as well as numerous sales partners worldwide.
Resources
[1] Recommendation ITU-T P.863: Perceptual objective listening quality assessment, 03/2018.
[2] ETSI TS 103 281: Speech quality in the presence of background noise: Objective test methods for super-wideband and fullband terminals, V1.3.1, 05-2019.
[3] ETSI TS 103 224: A sound field reproduction method for terminal testing including a background noise database V1.4.1, 08-2019.
[4] ITU-T Recommendation P.800: Methods for subjective determination of transmission quality, 08/1996.
[5] Pusch, A. et al., “Binaural listening effort in noise and reverberation,” in Fortschritte der Akustik DAGA 2018, volume 44, pp. 543–546, Berlin, Germany, 2018.
[6] Rennies, J. and Kidd, G., “Binaural listening effort in noise and reverberation,” in Fortschritte der Akustik - DAGA 2018, volume 44, pp. 615– 616, Berlin, Germany, 2018.
[7] ETSI TS 103 558: Methods for objective assessment of listening effort, 11-2019.
[8] ITU-T: Handbook on Telephonometry, ITU, 1992, ISBN 92-61-04911-7.
[9] ITU-T: Practical Procedures for Subjective Testing, ITU, 2011.
[10] Sottek, R.: Modelle zur Signalverarbeitung im menschlichen Gehör, Ph.D. thesis, RWTH Aachen, 1993, Techn. Hochsch., Diss., 1993.
[11] Sottek, R.: “A Hearing Model Approach to Time-Varying Loudness,” Acta Acustica united with Acustica, 102(4), p. 725–744, 2016, ISSN 16101928.
[12] Wan, R., Durlach, N. I., and Colburn, H. S.: “Application of a short-time version of the Equalization-Cancellation model to speech intelligibility experiments with speech maskers,” The Journal of the Acoustical Society of America, 136(2), pp. 768–776, 2014, doi:10.1121/1. 4884767.
[13] Durlach, N. I.: “Binaural signal detection: Equalization and cancellation theory,” Foundations of Modern Auditory Theory, Vol. 2, 1972.
[14] ISO/DIS 532-1, Acoustics – Reference zero for the calibration of audiometric equipment – Part 7: Reference threshold of hearing under free-field and diffuse-field listening conditions, International Organization for Standardization, 2005.
[15] Friedman, J. H.: “Multivariate adaptive regression splines,” Ann. Statist, 1991.
[16] Reimes, J.: “Auditory Evaluation of Receive-Side Speech Enhancement Algorithms,” in Fortschritte der Akustik - DAGA 2016, DEGA e.V., Berlin, 2016.
[17] Reimes, J. and Gierlich, H.W.: “Auditory Listening Effort Assessment for ICC systems,” Contribution to ITU-T SG12/Q4, T17-SG12-C-0157, 2018, Geneva/CH.
[18] Recommendation ITU-T P.581: Use of head and torso simulator for hands-free and handset terminal testing, 02/2014.
[19] Recommendation ITU-T P.56: Objective measurement of active speech level, 12/2011.
[20] Recommendation ITU-T P.1100: Narrowband hands-free communication in motor vehicles, 01/2019.
[21] Recommendation ITU-T P.1150: In-Car communication audio specification, 01/2020.