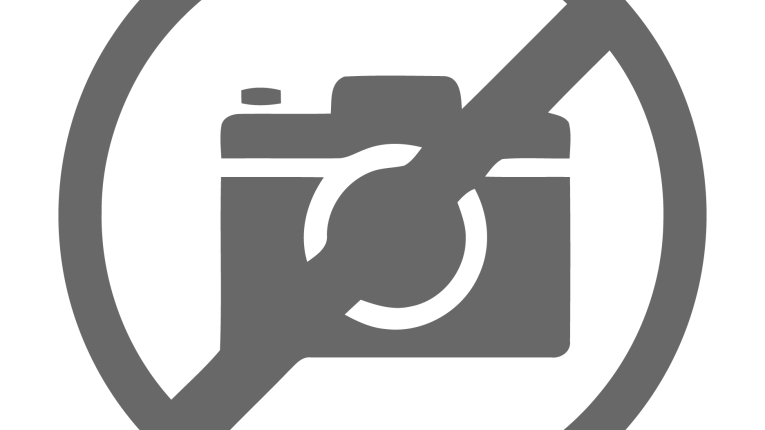
Synonymous with edge AI, TinyML has been described by Harvard researcher Matthew Stewart, PhD., as “the intersection of machine learning and the embedded internet of things (IoT) devices.” The field of TinyML grew from the concept of IoT, which traditionally operates under the assumption that data will be sent from devices to the cloud for processing. This method now raises concerns across enterprises about data security, latency and storage, energy efficiency, and connection dependency. In contrast, devices on the edge process data locally, taking the cloud and data centers out of the equation (Figure 1).
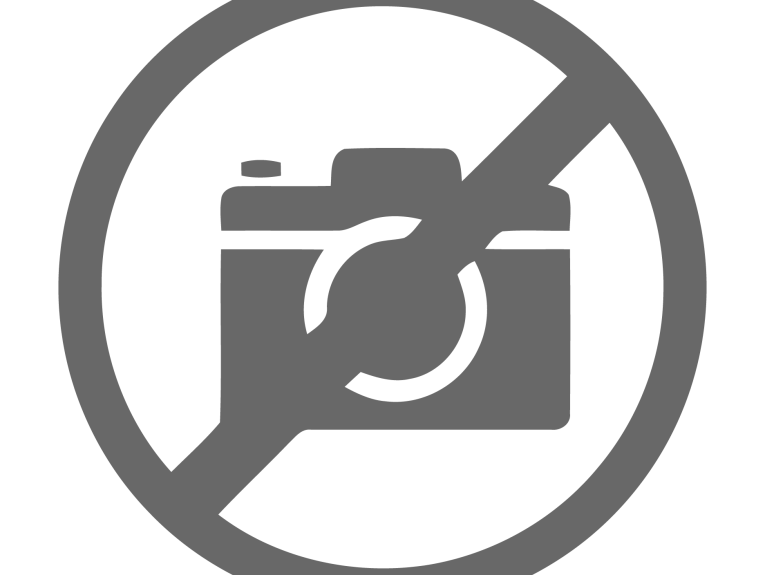
At the forefront of this evolution, usage of TinyML technologies and applications, specifically those utilizing voice, is growing as demand for specialized edge AI devices across industries and consumer spaces rises.
Evidence of this increased focus on low-latency, power-efficient edge AI technology capable of addressing the concerns caused by dependency on the cloud is ABI Research’s expectation that a total of 2.5 billion devices will be shipped with a TinyML chipset by 2030.
How TinyML Solves the Pain Points of Current ML Technology
Machine learning (ML) was defined in the 1950s by AI pioneer Arthur Samuel as “the field of study that gives computers the ability to learn without explicitly being programmed.” As modern machines are expected to handle more complex tasks ranging from language translation to powering autonomous vehicles and beyond, data storage and programming becomes more complex.
ML begins with data and from there programmers select a machine learning model to use, supply the data, and allow the computer to find patterns or make predictions. The more data a machine is able to train off of, the better the program, and programmers can change model parameters over time to push the machine to generate more accurate results.
Traditional ML technology has been trained to process large volumes of information to complete a variety of complex tasks and has a dependency on servers or the cloud for mass data storage, raising issues in enterprise and consumer spaces ranging from security to latency and energy efficiency.
Privacy concerns are front and center for connected devices (e.g., smart home appliances and voice assistants) that are always listening for a wake word in a user’s home. Concerns regarding privacy and compliance are enhanced when such devices are used in enterprise settings and secure information may be transmitted. On the user side, even small amounts of lag can alter an experience when interacting with smart TV remotes or wearable devices (e.g., fitness trackers or headphones). In an industry setting, high latency negatively affects efficiency. Energy efficiency is also key in AI-enabled devices to avoid the need for constant recharging or docking as most consumer devices are battery-dependent.
TinyML and Edge AI Technology Offer a Solution to Cloud-Dependent Devices
Low Latency/Offline Execution: Devices on the edge work offline and provide end users the convenience of operating their devices without an Internet connection. Cloud-free models ensure devices can run offline in (almost) real-time as there is no need for a round-trip latency that could be slowed by network connectivity levels.
Data Security: Data processed locally on the device does need to be sent to the cloud for processing or stored on servers. As concerns grow over privacy and smart devices are increasingly deployed in sectors that handle confidential information such as hospitals, offices, or factories, this factor becomes more relevant.
Energy Efficiency: Processing real-time data on the edge not only protects privacy and enables low latency, but also saves power. Edge AI is designed to have a low footprint and work on battery-powered devices for years at a time.
Despite recent developments in ML, finding an optimal solution for a given task remains challenging and time-consuming. It often requires significant effort in designing and tuning the neural architectures, particularly for embedded TinyML solutions, which need specific models due to limited computational resources.
One of the newest innovations in TinyML addressing this issue, Guided Neural Architecture Search with Imitation Learning (GNASIL), uses a ML tool called a soft-actor reinforcement learning (RL) agent to discover new neural networks quickly and effectively. It combines deliberate planning with predictions of the RL agent and chooses the best action at a time. In GNASIL, the reward function combines the accuracy of the newly discovered neural network structure on a validation set and the computational footprint of the designed architecture in floating-point operations per second (FLOPS).
Experiments on a series of on-device speech recognition tasks demonstrated GNASIL is performing as intended (Figure 2). The innovation can design neural models with competitive performance both in terms of discovery speed and the accuracy of the discovered architectures, all within the predefined (FLOPS) restrictions, helping design and optimize neural network architectures more quickly than before.
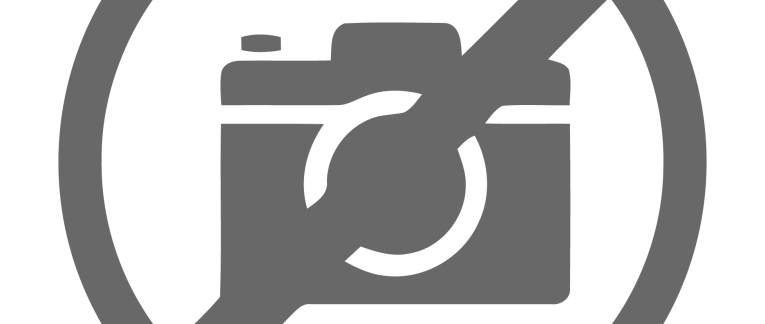
Deploying TinyML for the Enterprise
At the intersection of ML and IoT, TinyML is actively transforming enterprises as we know them. McKinsey researchers predict IoT will have a potential total economic impact of as much as $11 trillion by 2025, and Gartner expects the number of worldwide IoT-connected devices to increase almost threefold from 2018 to 43 billion devices by 2023. Combine these projections with the aforementioned expectations of ABI Research and the effect is a sector of the industry that “extends beyond the borders” of traditional technology.
The growth of TinyML has resulted in an increased interest in deploying speech and voice recognition devices across industries. TinyML hardware, algorithms, and software capable of performing on-device data analytics enable a variety of always-on use cases, targeting battery-operated devices in an array of industries from manufacturing to healthcare.
European manufacturer BSH deployed Fluent.ai’s offline, low-latency speech-to-intent AI technology in one of its German factories, estimating up to 75% to 100% efficiency gains on the assembly line due to voice automation.
Conventionally, factory operators must press physical buttons at their workstations to move appliances along the assembly line. To streamline efficiency and improve ergonomics, Fluent.ai developed a voice-control solution for workers to operate heavy machinery by speaking into a headset that is connected to an embedded voice recognition solution. Initial implementation of the solution cut down the transition time between stations by more than 50%.
Designed with loud factory settings in mind, the technology is robust and has the ability to cancel out background noise. It is also multi-lingual and accent-agnostic, allowing the technology to be deployed anywhere, regardless of location or spoken language.
In healthcare, Edge Impulse and Arduino applied an optimized TinyML model to build a cough detection system that runs in real-time in under 20kB of random access memory (RAM) in response to the COVID-19 pandemic. Further TinyML concepts have been designed that could analyze a patient’s heart rate, body temperature, and respiratory rate to predict a patient’s health condition.
Hardware partners such as Dell are also supporting TinyML with specialized hardware that hosts and integrates intelligent data at the edge, bringing innovation to IT and business stakeholders. It not only assists in delivering an intelligent insights platform for organizations, but also supports 5G and WiFi 6 innovations.
TinyML and the Future of Connected Devices
Achieving high efficiency on low power is the ultimate benefit of TinyML devices and innovations, such as GNASIL, are laying the groundwork for more optimized models. Within five years, voice recognition technology on the edge is going to permeate everyday life from smart appliances to wearables and office meeting rooms to restaurants as speed, convenience, efficiency, and privacy are prioritized (Figure 3).
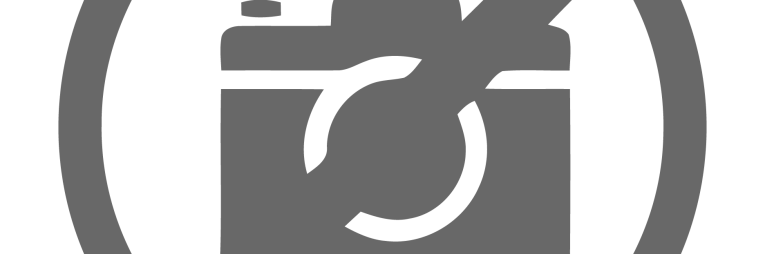
The industry is already seeing more specialized chips being designed, and tiny machine learning algorithms and low-power hardware (microcontrollers) have evolved to the point that it’s possible to run sophisticated models on embedded devices and speech technology is becoming the rule, not an exception.
Privacy and cybersecurity are two of the biggest concerns emerging from the surge of the voice recognition market and interconnectivity to the cloud, and TinyML devices are being designed to meet compliance obligations in consumer and enterprise settings. In the near future, specialized devices will be able to hear and alert when machines are malfunctioning or even when a faucet is leaking—all in an offline capacity.
Traditional speech understanding solutions operate in two distinct steps:
Speech is transcribed into text in the cloud. Natural language processing is applied to the text to determine the user’s intent. Cutting-edge voice recognition technology directly maps a user’s speech to their desired action without the need for speech-to-text-transcription or a cloud connection. A text independent approach enables the development of speech recognition systems for any language or accent that run completely offline and work accurately in noisy environments.
At the forefront of speech-to-intent technology, Fluent.ai employs unique deep neural network algorithms to map speech. The technology learns by directly associating semantic representations of a speaker’s intended actions with the spoken utterances (Figure 4).
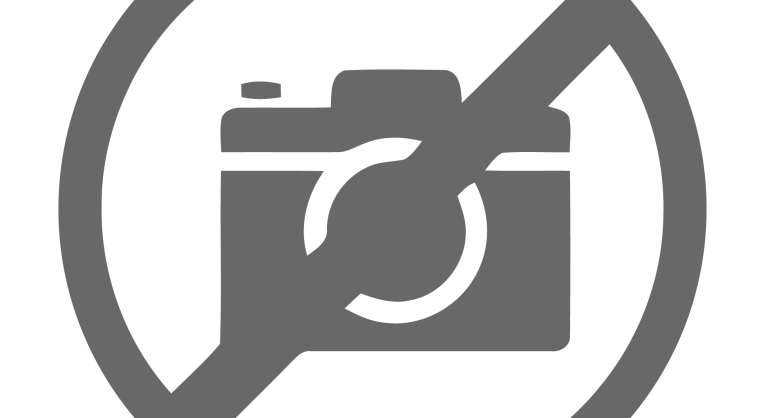
The current AI behind the technology is being trained to better understand accents from conception, and challenges from background noise are being mitigated to build more robust speech recognition experiences. The models are based on the concept of vocabulary and language acquisition in humans.
A text-independent approach enables the development of speech understanding models that can learn to recognize a new language from a small amount of data compared to cloud-based systems and enables end users to interact with their devices in a more natural and intuitive way.
Embedded voice solutions support an end user’s freedom to travel anywhere and do anything while protecting privacy and keeping their device’s smart voice capabilities with them wherever they go. From smartwatches and fitness trackers to headphones and earbuds, voice technology on the edge gives users the flexibility and hands-free convenience of accurate voice command control in any environment. Noise robust solutions also allow for usage in the noisiest locations, whether it be a busy street or a family gathering.
Highly portable and light footprint, offline speech recognition solutions are the technology driving today’s TinyML voice recognition demand surge. Systems capable of running on microcontrollers and digital signal processors (DSPs) will lead the evolution of voice recognition on small devices.
Voice has become a mainstream tool for enterprises, apps, and digital platforms due to its ability to simplify the user interface. As processes continue to be streamlined because of advancements in TinyML and voice recognition solutions, offline devices capable of executing specialized commands are going to permeate every industry. VC
This article was originally published in audioXpress, February 2022.
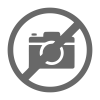
Vikrant Singh Tomar is Founder and Chief Technology Officer of Fluent.ai, Inc. He is a scientist and executive with more than 10 years of experience in speech recognition and machine/deep learning. He obtained his PhD in automatic speech recognition at McGill University, Canada, where he worked on manifold learning and deep learning approaches for acoustic modeling. In the past, he has also worked at Nuance Communications, Inc. and Vestec, Inc. as a Research Scientist.